Introduction
Recent advances in biomedical imaging have enabled the analysis of many different cell types. Automated cell detection is
an important field of interest with a wide range of applications which permits statistical analysis of various cell
parameters. However, learning based cell detectors tend to be specific to a particular imaging protocol and cell type.
For a new dataset, a tedious re-training process is required. In this paper, we propose a novel method of training a cell
detector that rapidly adapts to new datasets. First, the classiffication rules extracted from existing data are combined
with the training samples of new data using transfer learning. Second, a global parameter is incorporated to refine the
ranking of the classiffcation rules. Third, an active training method which selects the most valuable new training
samples first is employed to improve the detection accuracy. We refer to the proposed method as the Globally Active
Transfer Learning for Adaptive Boosting (GATLAB), a boosting-based transfer learning method that incorporates a global
parameter and active training to minimize the number of training samples. We demonstrate that our method achieves the
same performance as previous approaches with only 10% of the training effort.
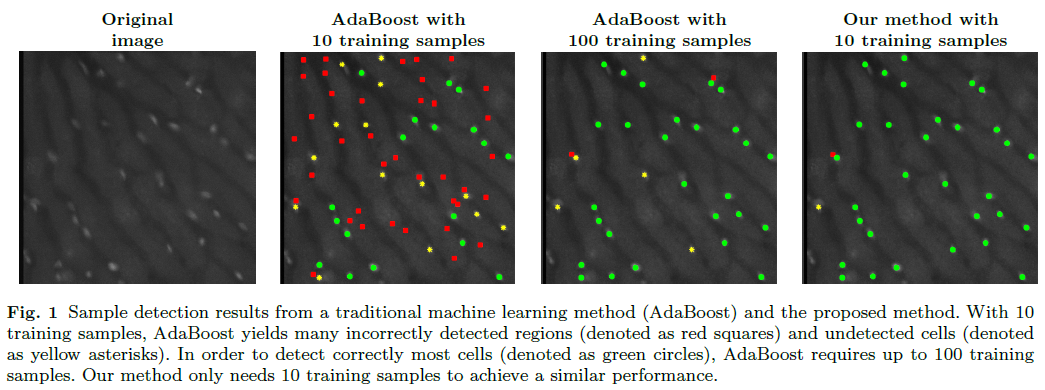
Data
The project is implemented in MATLAB. The program is free for non-commericial usage. We encourage other researchers to collaborate.
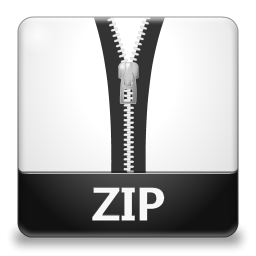
GATLAB Toolbox
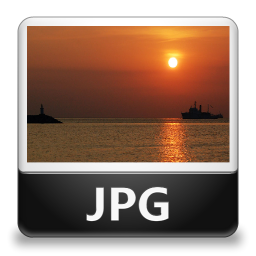
Data: original & results
Publications
- N. Nguyen, E.Norris, M. Clemens, M. C. Shin. "A Rapid Training Method for Cell Detection in Microscopy Images." Biomedical Engineering, IEEE Transactions on. Submitted Dec 2012. In Review.
- N. Nguyen, E.Norris, M. Clemens, M. C. Shin. "Rapidly Adaptive Cell Detection using Transfer Learning with a Global Parameter." Second International Workshop on Machine Learning in Medical Imaging (MLMI) In conjunction with MICCAI 2011, Westin Harbour Castle, Toronto, Canada. September 18-22, 2011. PDF Poster MLMI
People
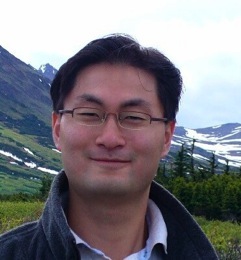
Min Shin, Ph.D. Associate Professor CS Department, UNC Charlotte Phone: (704) 687-8578
Office: 403D WOODW Email: mcshin at uncc dot
edu
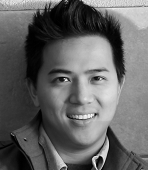
Nhat 'Rich' Nguyen, M.S. PhD Student
CS Department, UNC Charlotte Phone: (704) 687-8582
Office: 404 WOODWARD Email: nhnguye1 at uncc dot
edu
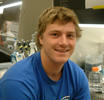
Erric Norris, M.S. PhD Student in Biology
Biology Department, UNC Charlotte Phone: (704)
687-8675 Office: 463 WOODWARD Email: enorris9
at uncc dot edu
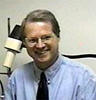
Mark Clemens Ph.D. Professor and Vice Chair of
Research Biology Department, UNC Charlotte Phone:
(704) 687-8682 Office: 486A WOODWARD Email:
mgclemen at uncc dot edu
This material is based upon work supported by the National Science Foundation under Grant No. DBI-0754748.