Software Releases
Research Talks
Conference Posters
Slides
Funding Agencies
Current Research
FloodWatch: A Data Platform to Predict Urban Flooding in Smart Cities
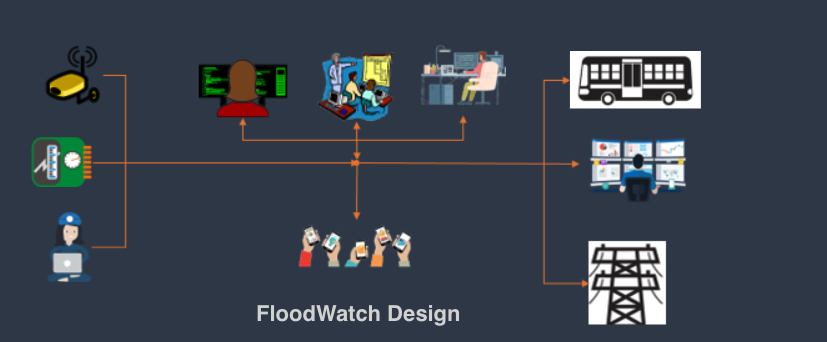
Fall 2021 -- present. Objectives of this project include developing a secured, distributed data infrastructure allowing data owners to share data effortlessly and securely, while concurrently allowing data users to access data on-demand. The data infrastructure is to underpin disaster response and decision support applications. The new focus on flood forecasting and alert is recommended by our collaborator in Vietnam. There is strong interest for this service since urban flooding is a regular challenge in HCMC. The collaborating team in Vietnam has expertise and experience in urban flood modeling; and they also have access to the necessary data (e.g., roads, drainage infrastructure, rain gauges, and weather forecast). Our project also partners with Ho Chi Minh City Department of Science and Technology. The recommended strategy is once we have developed a pilot app, our partner will convene a demo with city government agencies to get their buy in and input.
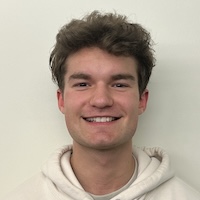
Alex Foster
Virginia '26
RA - Full-stack Lead
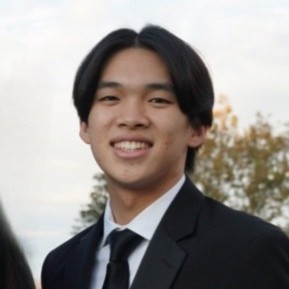
Andrew Ma
Virginia '25
RA - ML Lead
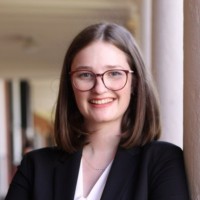
Natalie Dahlquist
Virginia '25
RA - IoT Lead
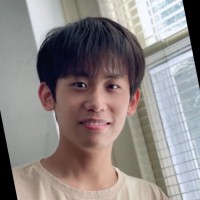
Han Yan
Virginia '24
RA - Full-stack
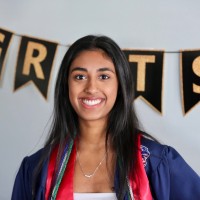
Nikhita Guntu
Virginia '24
RA - ML
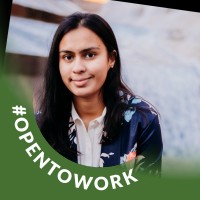
Nitya Khamar
Virginia '26
RA - IoT
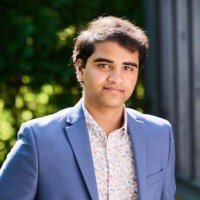
Vainateya Rangaraju
Virginia '25
RA - Federated Learning
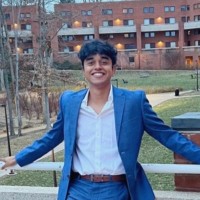
Niket Anand
Virginia '25
RA - ML
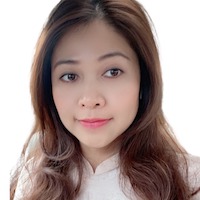
Anh-Thu Nguyen
Vice Dean, DNIIT
Collaborator at UD-DUT
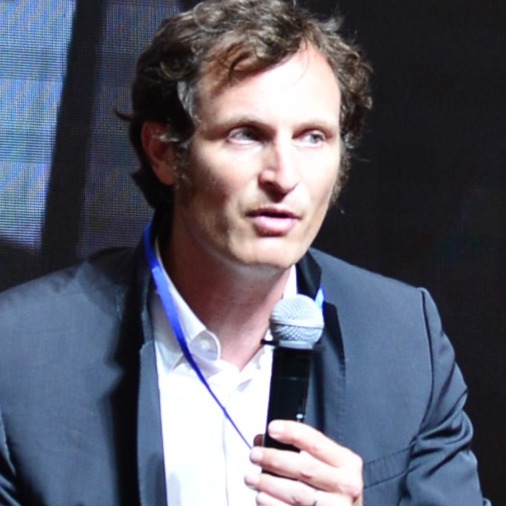
Fabien Ferrero
Professor of IoT
Collaborator at UCA
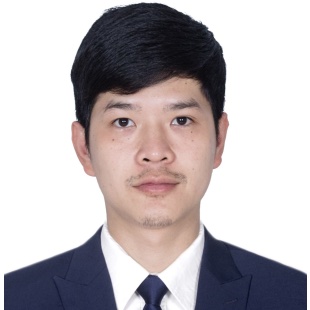
Huy Le Trinh
Assoc Prof of Embedded System
Collaborator at VNU-UIT
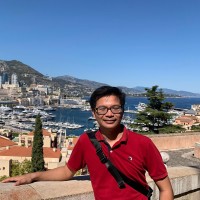
VanLic Tran
Software Architect
VN Galaxy
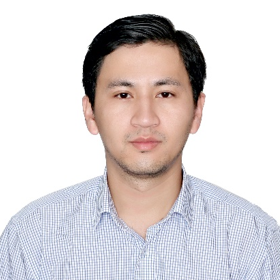
Khoi Dao
Assoc Professor of Environment
Collaborator at HCMUS
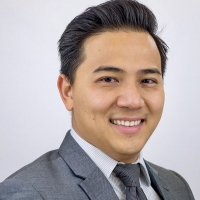
Rich Nguyen
Asst Professor of CS
PI
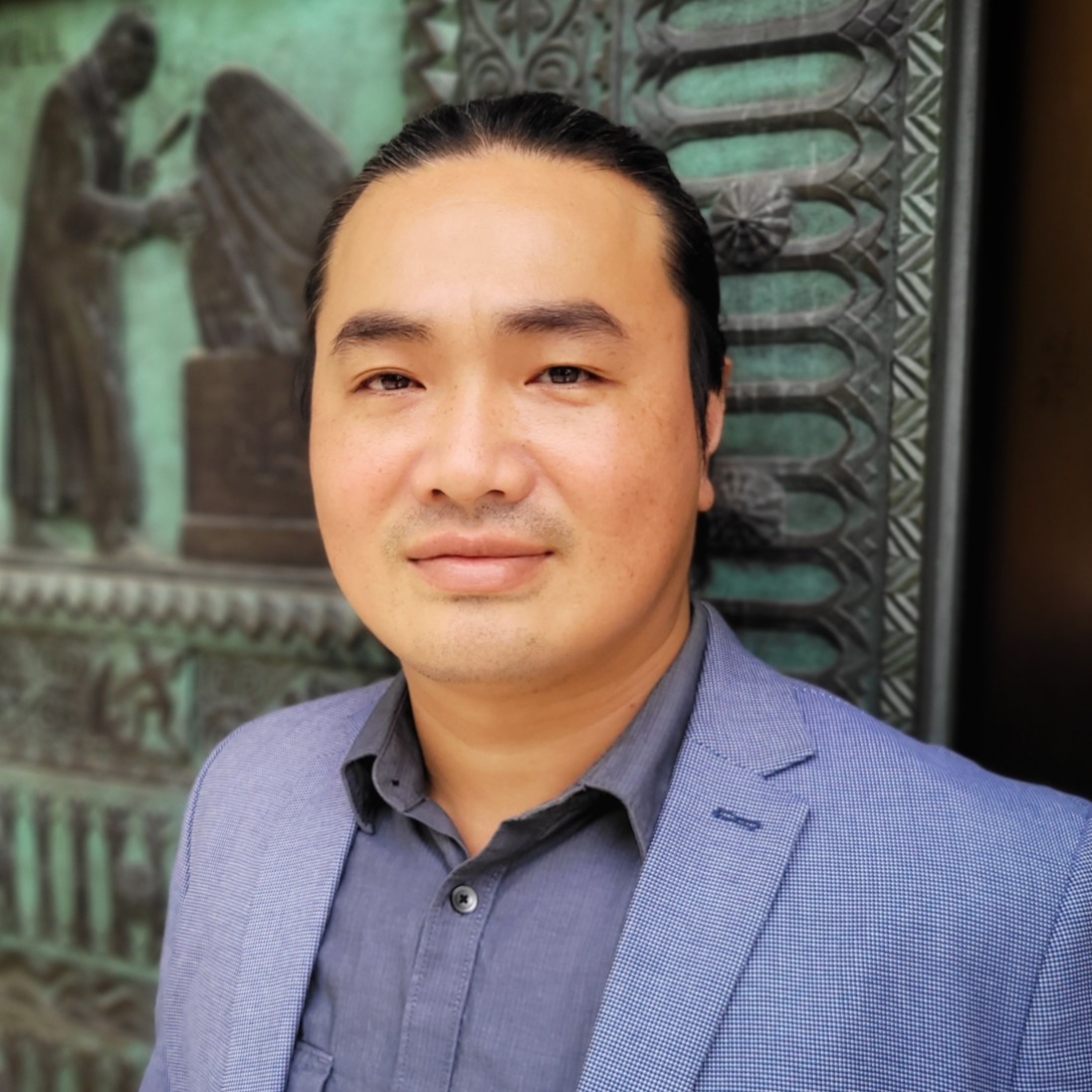
Tho Nguyen
Program Manager at NAS
Co-PI
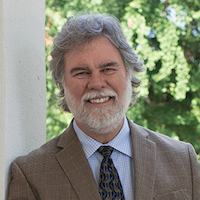
Ron Hutchins
UVA Vice President for IT
Co-PI
TuneScope: Computational Thinking Through Arts and Music
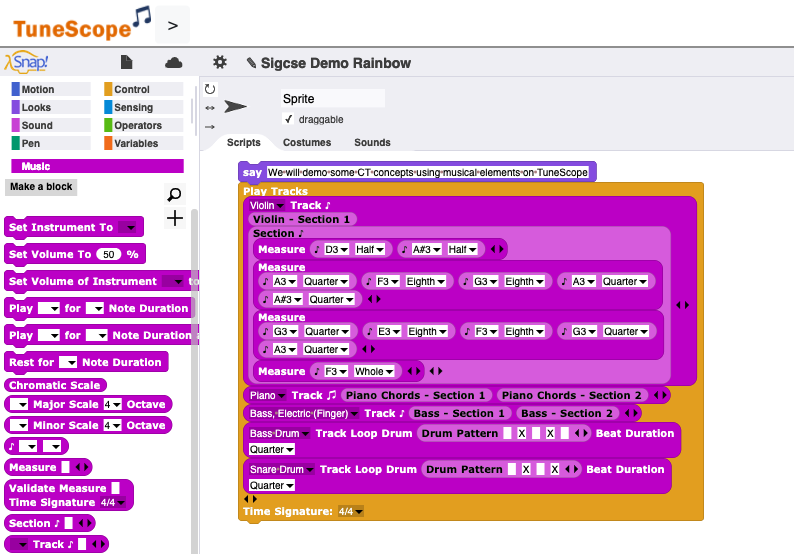
Spring 2019 -- present. Since 2016, the Commonwealth of Virginia requires that all schools must teach computer science and computational thinking, including computer coding. Virginia's Standards of Learning specify that these concepts must be integrated into content courses at the elementary level and taught as stand-alone courses in middle school and high school. TuneScope is a digital tool designed to create sound effects and music on a computer. TuneScope was developed through a collaboration between the School of Education and Human Services and the Department of Computer Science at the University of Virginia. It combines a sound analysis & synthesis tool with a computing language, Snap!, developed at the University of California at Berkeley. TuneScope extends Snap! by incorporating additional sound visualization and music synthesis capabilities.
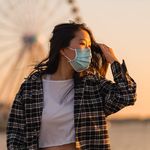
Janice Guo
Virginia '25
RA - Web Dev
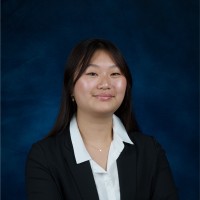
Cheryl Bai
Virginia '25
RA - Web Dev
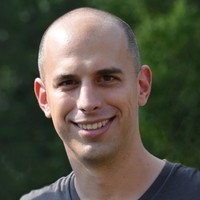
Jo Watts
MakeToLearn Lab Manager
Project Manager
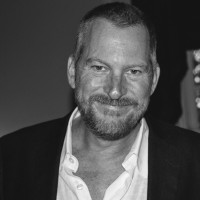
Monty Jones
Assoc Professor of Education
Consultant
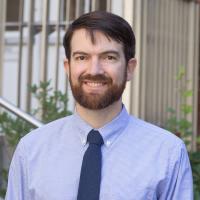
Luke Dalh
Assoc Professor of Music
Co-PI
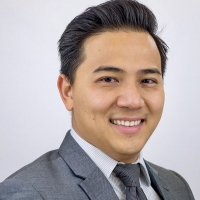
Rich Nguyen
Asst Professor of CS
Co-PI
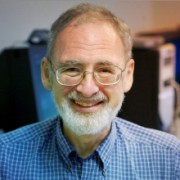
Glen Bull
Professor of Education
PI
Previous Projects
Deep Diagnosis: Deep Learning for early detection of Bloodstream Infection in Intensive Care
Spring 2020 -- Spring 2023. Sepsis is a syndrome of critical illness defined as life-threatening organ dysfunction due to a dysregulated host response to infection. It is the leading cause of global mortality causing approximately 1 out of every 5 global deaths. Bloodstream infections (BSIs) are a frequent cause of sepsis and are associated with a high mortality rate. Attempts to compose multivariable scoring systems and clinical prediction rules for BSI primarily involved categorical variables (e.g. heart rate >90 bpm or < 90 bpm), utilized relatively small data sets, and led to only moderate predictive efficacy. We propose to capitalize on the large amounts of continuous physiological data captured in the ICUs of multiple hospitals to develop stronger predictive models of BSI than previously published models. These models can learn extremely complex relationships between data inputs and outcomes, and have exceeded human abilities in performing classification tasks similar to identifying patients at risk for BSI.
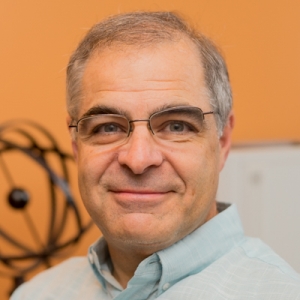
Giles Clermont
Professor of Medicine
Collaborator at Pitts
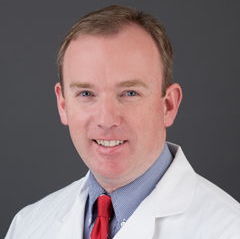
Chris Moore
Infectious Diseases Doctor
Co-PI
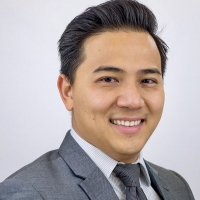
Rich Nguyen
Asst Professor of CS
Co-PI
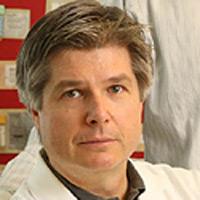
Randal Moorman
Professor of Medicine
Co-PI
UVA Historical Landmark Recognition using TensorFlow 2.0
Spring 2019 -- Spring 2020. The UVA Grounds is known for its Jeffersonian architecture and place in U.S. history as a model for college and university campuses throughout the country. Throughout its history, the University of Virginia has won praises for its unique Jeffersonian architecture. We have collected a large image repository and dataset on 19 buildings on UVA Grounds. Using those images as training data, we build an image recognition system to classify different buildlings/landmarks on Grounds.
CodeNC: Integrating Computational Thinking into K-12 Instructional Activities using Animated Videos
Spring 2018 -- Summer 2018. Increasing the representation of minorities in computer science (CS) has become a national priority. One of the many reasons minority students nationwide choose not to study CS is that they often lack mentors and role models to encourage them early in their learning. However, a big issue facing this initiative is that many K-12 teachers finds themselves lack of (1) relevant materials, (2) systematic training, and (3) a supportive community. The democratization of media, such as photos and videos, has provided a great variety of options to educate a broad audience on myriad topics. In this poster, we will describe the challenges and successes of using animated videos including its beauty, soundness, and utility as critical elements in establishing a strong CT comprehension while engaging K-12 teachers in a non-threatening way.
Affective Peer Tutoring: Fostering a Sense of Belonging among Female CS students
Spring 2016 -- Fall 2017. One of the many reasons female students nationwide choose not to finish their study in CS is that they do not feel a deep sense of belonging in the major. To foster the sense of belonging among female CS students, the affective learning outcomes, which are adapted from Bloom's Taxonomy on human learning, are integrated into the context of peer tutoring as five successive stages. Through the five stages of affective peer tutoring, students gradually deepen their sense of belonging in CS by: (1) being aware of the tutoring services; (2) proactively seeking answers to their programming questions; (3) recognizing the value of self-efficacy; (4) discussing learning issues in a supportive environment; and (5) contributing to a peer-led learning program to help others. Therefore, this poster may be of interest to any CS educator who wishes to improve the interaction, performance, and retention among female CS students while sustaining a peer-led learning program at their institution.
Detecting Social Insects in Videos
Spring 2015 -- Spring 2017. The studies of the network formed by social insects require the motion analysis of their interactions and movements in videos over an extended period of time. Automated detection is an important field of interest because it enables the motion analysis in large-scale experiments. When an automated detection method is applied to various insect types, the training task often involves the collection of a large number of labels provided by human experts. To save the experts' time and effort, unlabeled data have been recently employed to supplement the training. In this paper, we utilize the spatiotemporal connectivity of the unlabeled data to regulate the training of a detector on a new insect type.
Improving Pollen Classification
Spring 2012 -- Spring 2014. This research focus mainly on the automated classification of pollen using computer vision and machine learning. Automated methods still requires a large number of training samples as the number of pollen types increases over time. We reduce the training effort of the classification with three main key points: a modified transfer learning framework, a new active selection criteria, and a new spike count feature.
Rapidly Adaptive Cell Detection
Spring 2010 -- Spring 2012. Different imaging protocol and cell type result in various cell appearances. We present a novel method of training a cell detection method on new datasets with minimal effort. We show that our method reduces the training effort up to 10 times.
Tracking Colliding Cells
Summer 2007 -- Fall 2009. It's the first tracking algorithm for colliding cells. The motion of leukocytes is significant in studying the inflammation response of the immune system. We model the collision states of cells and testing multiple hypotheses of their motion and appearance.
Alum RAs / Research Capstones / Fellows
RA Contribution. I was so fortunate to lead a group of talented research assistants, capstone research students, and research fellows. Their energy, contribution, and hardwork have made my research projects relevant and impactful.
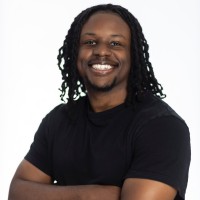
Chris Barfield
Virginia '24
SWE at Netflix
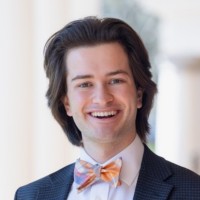
Sean Miller
Virginia '24
Consultant at Oliver Wyman
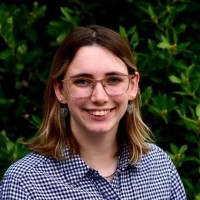
Emily Branch
Virginia '24
MS Student at UPenn
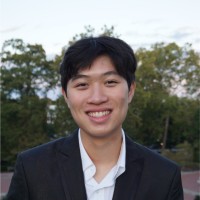
Daniel Huynh
Virginia '24
SWE at Ansys
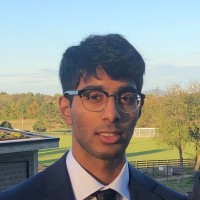
Sidhardh Burre
Virginia '24
SWE
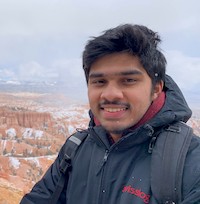
Abhir Karande
Virginia '24
MS Student at UCLA
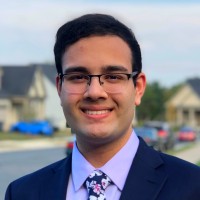
Param Damle
Virginia '24
MS Student at CMU
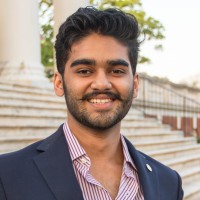
Rishi Mukherjee
Virginia '24
SWE
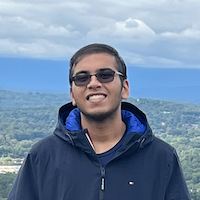
Ankit Gupta
Virginia '23
MS Student at CMU
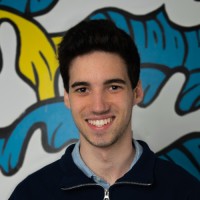
Chris Santamaria
Virginia '23
UX at Figma
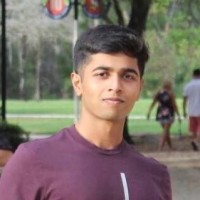
Harsh Padhye
Virginia '23
SWE at Appian
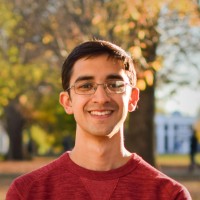
Katik Chugh
Virginia '23
SWE at Systems P&A
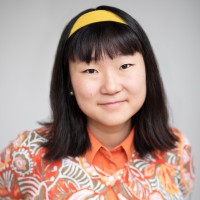
Rachel Gibson
Virginia '23
Teacher at C-ville High
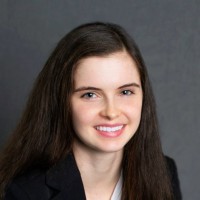
Louisa Edwards
Virginia '23
MS Student at Stanford
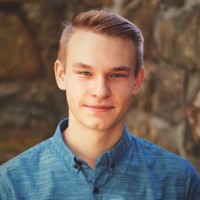
Zack Boner
Virginia '23
PhD Student at Duke
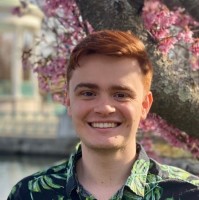
Jackson Brandberg
Virginia '20, CAMA
Data Scientist at State Farm
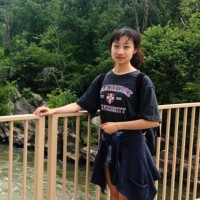
Joy Qiu
Virginia '21
Data Scientist at CAMA
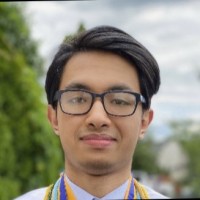
Shiva Manandhar
Virginia '23
Engineer at Veeva Systems
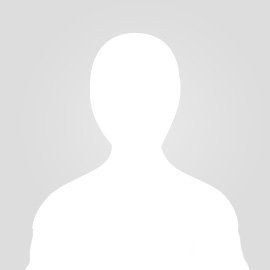
Shuo Yan
Virginia '23
MS Student at UMich
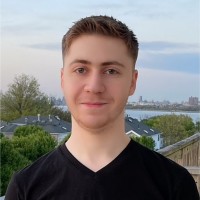
Eric Stein
Virginia '22
SE at Google
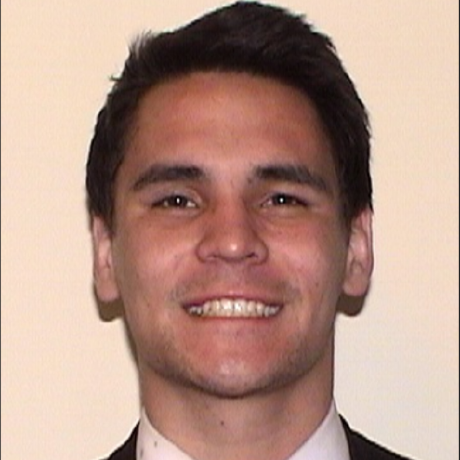
Ethan Gumabay
Virginia '22
SE at AWS
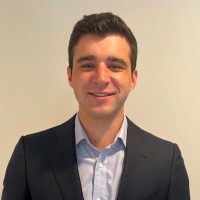
Matthew Pillari
Virginia '22
MLE at ImaGen
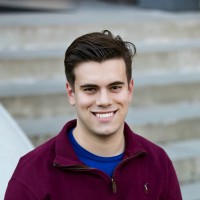
Christian Jung
Virginia '22
PM at Microsoft
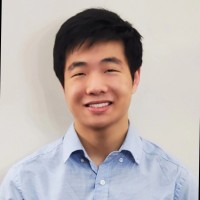
Adriel Kim
Virginia '22
SE at Microsoft
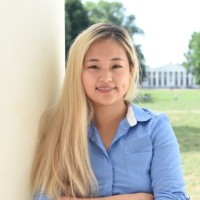
Annie Cao
Virginia '22
SE at TD Securities
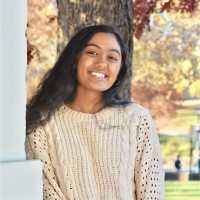
Medhini Rachamallu
Virginia '22
SE at Illumina
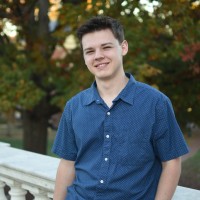
Preston Wright
Virginia '22
SE at Zoom
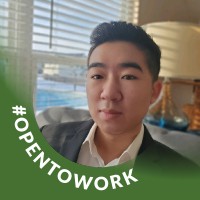
David Xue
Virginia '22
SE Intern at Amazon
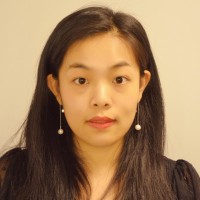
Yuxin Wu
Virginia '21
MS Student at UCLA
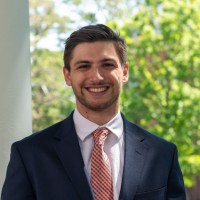
Navid Jahromi
Virginia '21
SDE at Palantir
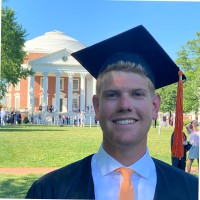
Adam Marcus
Virginia '21
SDE at Amazon
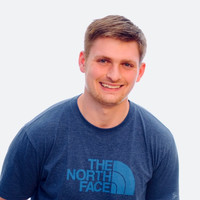
Arthur Harris
Virginia '21
RA at UVA
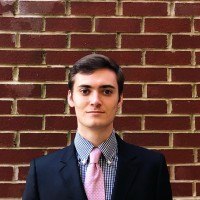
Nicholas Newton
Virginia '21
Data Scientist at SWIFT
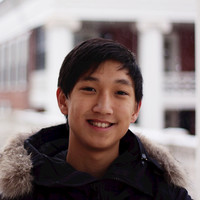
Cory Kim
Virginia '20
SDE at Amazon
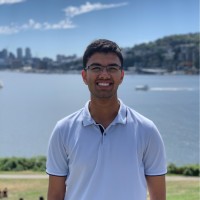
Siddharth Nanda
Virginia '20
SE at Microsoft
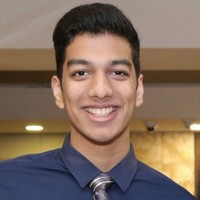
Siddhant Goel
Virginia '20
SE at Argo AI
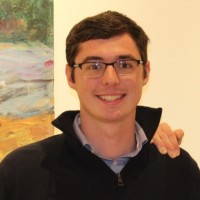
Mark Maguire
Virginia '19
CyberSec at Drangonfli
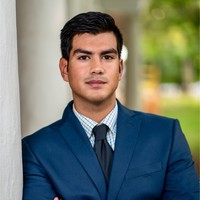
Jimmy Flores
Virginia '19
SDE at Amazon
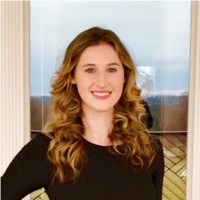
Kyra Ballard
Virginia '19
SE at Instagram
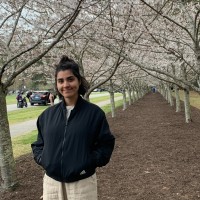
Parima Sahbai
Virginia '19
UX Designer at Adobe
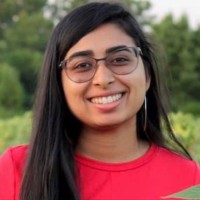
Tanvi Patil
Charlotte '20
Crown Scholar at Chapel Hill
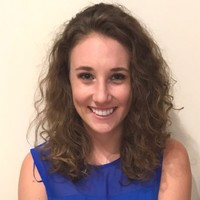
Natalie Lang
Charlotte '20
SE at Wells Fargo
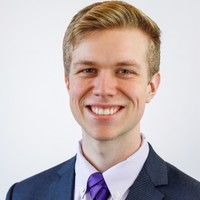
Logan Willett
Charlotte '20
SE at Fidelity
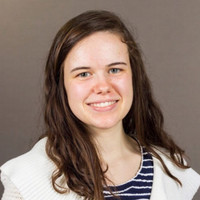
Hunter Healy
Charlotte '20
CyberSec at Visa
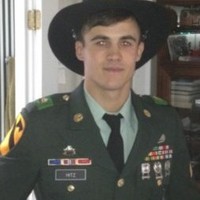
Thomas Hitz
Charlotte '20
Security Admin at JSCM
Sahithi Meduri
Charlotte '20
Security Engineer at AWS
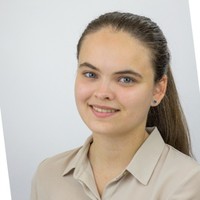
Iuliia Poliakova
Charlotte '19
Student at Chapel Hill
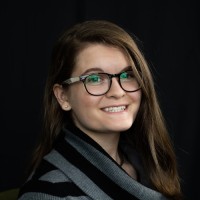
Hannah Hutcheson
Charlotte '19
PM at MUFG
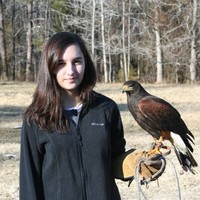
Jennifer Daly
Charlotte '19
QA at Guild Morgage
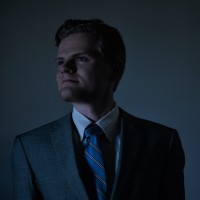
Joshua Hutcheson
Charlotte '19
SE at SealedAir
Current and Past Sponsors
Acknowledgement. This material is based upon work supported by the School of Engineering and Applied Science at the University of Virginia, which is supported by the National Science Foundation (under Awards #1842342 and #2026050) and generous fundings from UVA GIDI, UVA 3Cavaliers, Charlotte CCI, and Google.
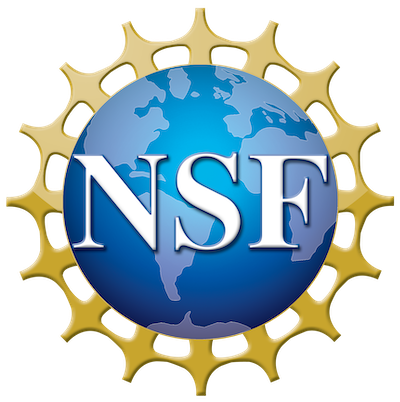
NSF Division of Research on Learning
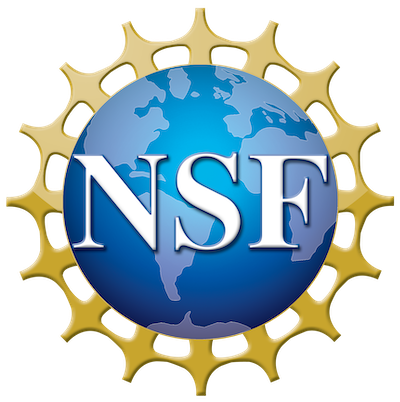
NSF Division on Computer and Network Systems
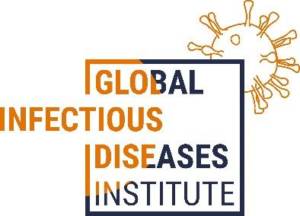
UVA GIDI
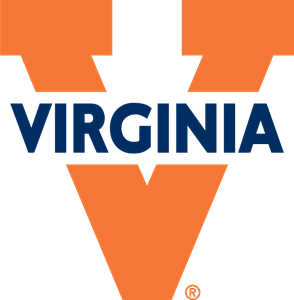
UVA 3Cavaliers
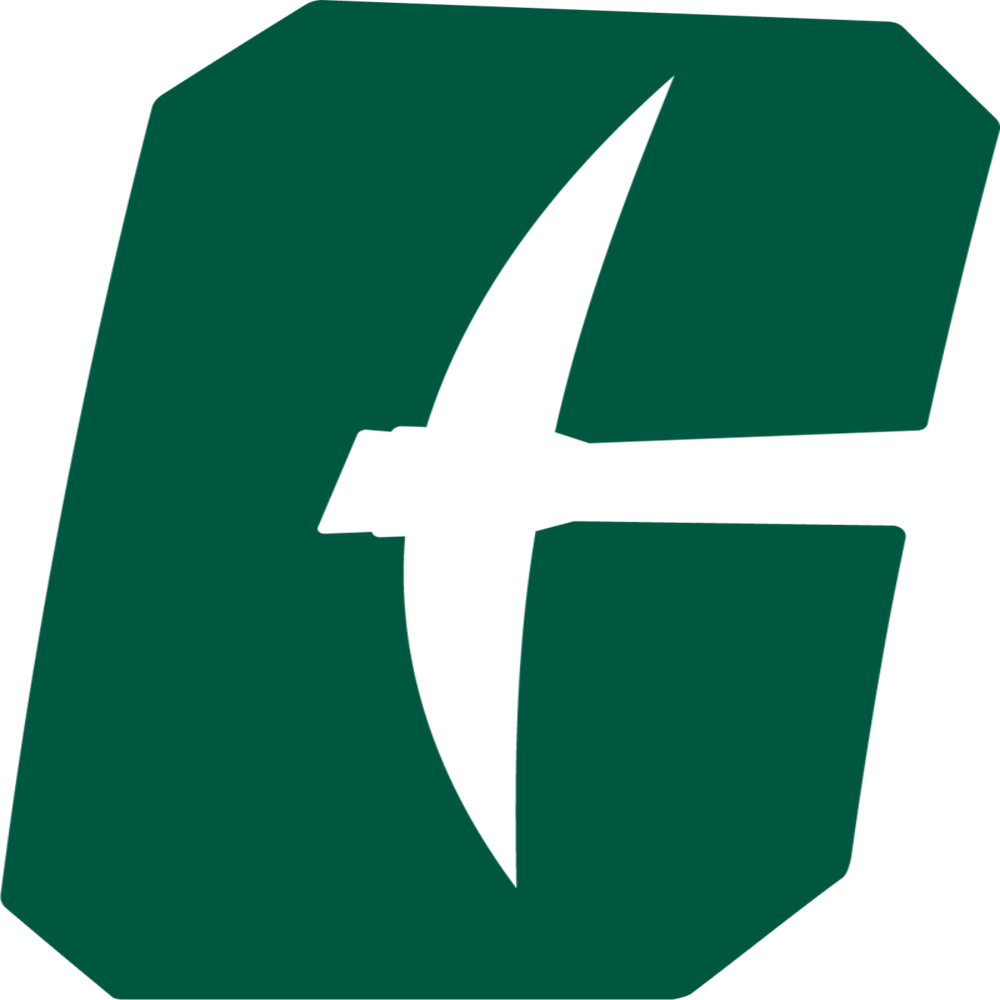